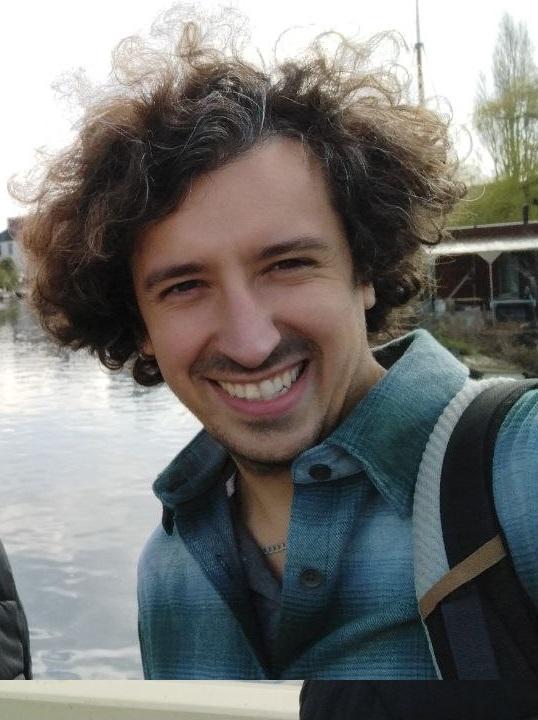
I am enthusiastic about interdisciplinary research. In my studies of mechanical (BSc) and acoustical engineering (MSc) I focused on topics of fluid mechanics and digital signal processing. I was able to apply my engineering skill set to bioacoustics for the first time during my master’s thesis, in which I developed a deep learning model for North Atlantic humpback whale song. Combining digital signal processing and machine learning to apply it to animal vocalizations sparked my excitement and got me thinking about continuing research in a PhD project. I am grateful to be working at the Naturalis Biodiversity Center and hope to use this opportunity to ensure that the technological advancements that we develop are distributed to practitioners and conservation organizations on the ground.